3 reasons your colleagues don’t want to use data—and how to change their minds
Part one of a two-part series on data adoption
April 7, 2023, By James Cousins, Senior Strategic Leader, Data and Analytics
Has this ever happened to you? Your office spent weeks collecting data, building reports, and setting up a new dashboard for your colleagues. Several months down the road you look at the adoption rates, only to discover they are less than you had hoped.
Or perhaps this sounds more familiar: You’re in yet another meeting where one voice exclaims, “We need data!” only to have all other voices fall silent when someone asks for volunteers to actually investigate the data. The causes may vary but the end result is the same: despite significant efforts, some of your colleagues just won’t use data.
As a strategic leader for Rapid Insight, I spend most of my days working with IR staff and other data analysts to build impactful predictive models, create useful dashboards, and help them conduct high-impact analyses. As such, I often hear the analyst’s perspective on frustration about low adoption.
Below is what I’ve learned from those conversations. Read on for three common reasons people don’t want to engage with data, and ideas to win them over.
Three Reasons Your Colleagues Don’t Use Data
Reason 1: A “That’s Not My Problem” Mentality
Supporting a student through the entirety of their experience takes a village, as the saying goes. It’s hard to argue that any facet of the student’s career is isolated from the other facets. While most staff and faculty would agree with that sentiment, some may feel that they either have nothing to add to data initiatives or don’t need to concern themselves. The key is to help colleagues recognize that everyone has a role to play in data analysis.
Solution: Creating Strategic Partnerships
In our experience, it’s a fair bet that the perception of data falling “outside” of a department’s concerns comes down to thus-far-unearthed common ground. Wherever we are in our data project—collection, validation, analysis, modeling, reporting, or otherwise—we often think only of how it benefits our direct stakeholders. But as analysts, we’re well suited for the lateral thinking that might be required to help indirect stakeholders see that our work really does merit their interest.
Case Study
Longwood University sought to improve student retention using predictive models. They built a predictive model that provided an estimation of risk that an incoming student would end up on academic probation within their first semester. But many of us who have performed a similar analysis know that having the data does not immediately translate to actions that impacts change. To operationalize the insights, the analyst at Longwood formed a partnership with their librarians. A proactive librarian mentor program became a popular way to orient and support students at the institution, even expanding beyond the initially identified risk pool!
Reason 2: Failing to Recognize Opportunity Cost
With the number of evergreen challenges that any institution is facing (e.g., getting the right class size, improving retention rates) it can be difficult to generate momentum for any non-essential new initiative. “Non-essential” is an important qualifier—resistance from peers along the lines of “Why do more?” stems from the estimated work outweighing the perceived value.
In those cases, it falls on data practitioners to clearly convey what they’ve learned from the data. If what’s currently in place is working sub-optimally and stakeholders are resisting opportunities for process improvement regardless, you may need to communicate the value in other terms.
Solution: Focus on Gaps in the Status Quo
The key is to approach your analysis or your presentation in a way that makes the cost of inaction highly visible to your stakeholders. Sometimes, you’re able to choose the topic of your analysis, so you can avoid stakeholder ambivalence by focusing on a problem your colleagues have already acknowledged. That might mean prioritizing a smaller problem that is mutually recognized by your stakeholders over a relatively larger one that isn’t. Other times, you’re unable to choose the topic, so you can only change how you present your analysis. Be sure to present metrics that will speak to them. If they aren’t as compelled by the issue you’ve discovered, they may be missing a dimension of the problem. Focusing on challenges and presenting findings that are more pressing to your partners is an effective way to establish or increase your reputation as an office and individual.
Case Study
Lincoln Memorial University’s DeBusk College of Osteopathic Medicine (LMU DCOM) introduced a new workflow for advisors to access student grades. Getting support to change a business process is hard whenever most of the inefficiency is your problem more than theirs. However, by emphasizing that the existing method of accessing student grades was translating to reporting latency and missed intervention opportunities at key student milestones, LMU DCOM’s advisors alike saw the value in adopting the new, streamlined method the analyst recommended.
Reason 3: A Lack of Communication
Mutual communication is when analysts welcome stakeholders’ thoughts and subject matter expertise, and stakeholders take the time to empower their analysts with background rather than sparse requests. Whether the data practitioner is failing to consider the value of stakeholders’ context or the stakeholder fails to see the value of providing it, this lack of communication harms the relevance of the final product. It’s important not to skip or shorten the “discovery” phase of your analysis because of how many strategic connections can form during it.
Over time, instances where datasets, dashboards, and predictive models miss the mark can lead to ambivalence and decrease confidence in data. Thus, there are two factors to consider when you observe low adoption of your insights: do you really understand their needs? And do they feel like you understand their needs? Both of these factors are risks when you have a lack of mutual communication.
Solution: Inclusivity in Analytics
Determining the signal from the noise is the goal of data analysis. We often develop reliable instincts that tell us what really matters. All professionals develop subject matter expertise as they gain experience though, and as discussed earlier, data analysis isn’t really just about data. It has context, and our conclusions have consequences. Thus, it pays to be inclusive throughout your analysis. A dramatic unveiling of predictive scores or a new dashboard is exciting, but it may be counterproductive when you need to revisit earlier steps and address stakeholder’s concerns.
Bringing your stakeholders into the process is an easy and informative way to avoid red-tape at the tail end. We’ve seen several strategies work well:
- Start with a discovery session
Whether you’re overhauling a dashboard, building a model, or assessing an institutional service’s effectiveness, hosting a conversation to inventory the current setting helps. You may hear about go-to resources your stakeholders reference that you didn’t know about. You might hear about a pain point that you want to pay close attention to in your upcoming analysis. At the very least, you will find that your audience isn’t surprised when you deliver them a report later on!
- Offer updates when your work has phases
Analysis is not always a linear path. Data can surprise us with errors just as easily as it can with new and interesting questions. When your work spans enough time, updates can be a good time to share progress. More interestingly, it could be a good time to reincorporate their subject matter expertise into what you’ve learned or generated so far.
- Empower stakeholders who are especially good allies
The entire process of including colleagues in your work isn’t simply a way to facilitate your own delivery. It’s also an incredible way to stay deeply in touch with the priorities of other departments. When you find a stakeholder who seems to have a penchant for data—maybe they ask great questions, they spot fine details, or perhaps they’re just very good at operationalizing findings—do yourself the favor of keeping them in the habit!
In Conclusion
We’ve just explored three reasons why your colleagues might not want to engage with data. Hopefully by enacting some of these strategies you can get your team on board to start making more data-informed decisions. What do we do if our colleagues want to use data but still aren’t leveraging available solutions? In the next part in this series, we will explore six of the most common reasons why data-hungry colleagues don’t engage with data and analytics.
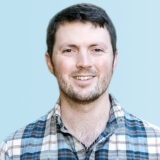
More Blogs
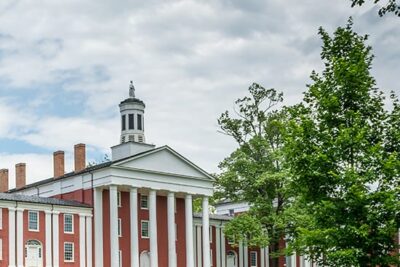
Creating a data-informed campus: part 1
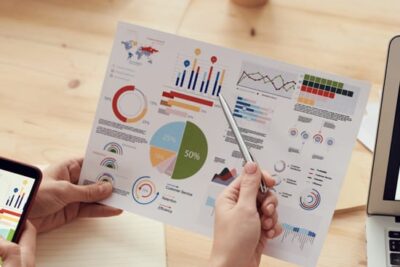
Where are the gaps in your data strategy?
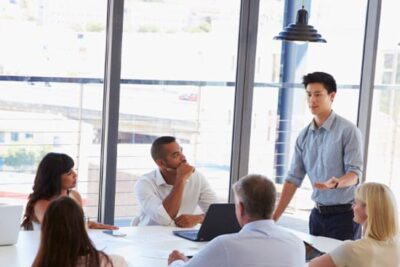