Creating a data-informed campus: part 3
Using data to facilitate institutional effectiveness
January 30, 2023
The conversation around data-informed decision making in higher education continues to accelerate. In the life of a campus information technology or institutional research professional, rarely a day goes by that data is not positioned as a universal solution, whether by a vendor or the higher education media. All too often, however, the question of how to capture these data and use them to positively affect the institution remains.
In the first blog post in this series, I discussed the necessity of a sufficient technology infrastructure to facilitate curation, access, and retrieval of data. In the second, I examined how to develop, structure, and staff an analytics organization. In this third and final post, I turn our discussion to the utility of the analytics function and how it can achieve real institutional impact.
3 key functions of a higher education analytics organization
Institutions of higher learning are collecting reams of data, but these data are generally used to satisfy compliance reporting requirements (or remain unused) rather than employed to facilitate strategic analysis (1). Used properly, data can help to facilitate the goals and growth of an organization and can help leaders and stakeholders better identify and understand areas of concern-and areas of success. Data integration, governance, and visualization are primarily focused on developing an infrastructure that can deliver analytics. Developing an effective analytics organization is the next step to actually use these tools and foster data-informed decision making across the institution. Here are three key functions of an effective analytics organization-and why developing one should be a focus area for every higher ed leader.
1. Meet increased calls for accountability
Calls for increased accountability in higher education permeate the campus climate at both public and private institutions. Accountability comes in many forms: to state or governing agencies, students, key performance indicators, operating measures, and more. A common component of accountability is an increased reliance on data to inform decision-making. Demands for new systems of accountability have resulted in an explosion of interest in data-driven decision-making. Indeed, “one of the defining characteristics of current U.S. educational policy is a focus on using evidence, or data, to inform decisions about institutional and educator quality, budgetary decisions, and what and how to teach students” (3).
Performance-based funding
Performance-based funding schemes operationalize accountability by incentivizing institutions to meet established goals. In these funding schemes, “…the relationship between performance and funding is predetermined and prescribed: if an institution meets a specified performance target, it receives a designated amount or percentage of state funding” (5). Completion agendas from outside entities such as Complete College America, the Bill & Melinda Gates Foundation, the Lumina Foundation, and others have further promoted the use of this funding mechanism (2).
In this “age of accountability,” data and analytics will only become more important to address, analyze, and communicate institutional performance to both internal decision-makers and external stakeholders. By using data to accurately assess institutional successes and challenges, the accountability discussion can become more illuminating and nuanced.
4 common barriers to data and analytics success
2. Create a data culture
Developing a “data culture,” where data use is embedded throughout the organization, is critical to effective data-driven decision-making (8). The shaping of an institutional “data culture” or evidence-based climate can be heavily influenced by the academic leader. Compared to other staff, academic leaders “…have more opportunities to leverage and regulate behavior by shaping what is valued or discounted and what is privileged or suppressed” (6).
An effective leader must also focus the organization on outcomes and improvements suggested by the data-rather than focusing on the data itself. Processes that are too data-intensive or bureaucratic may actually hamper strategic thinking. This was particularly true as I architected institutional effectiveness functions at prior institutions. For example, academic assessment processes were designed to comply with the reporting requirements of accrediting organizations. Although these requirements are unavoidable burdens, I worked with faculty committees to identify data that would also be usable by them as they educated students. Together, we were able to review available data, identify data that could inform institutional improvement, and design assessment systems that met not only compliance obligations but added strategic value to the institution.
Strategic planning and strategic thinking are both necessary parts of an effective data culture. Leaders should “suggest a dichotomy wherein strategic planning is data-driven information processing and strategic thinking is creative imagination” (9).
3. Elevate institutional research
Professional experience and knowledge help to shape the intentional, deliberative reflection on data (3). Institutional research “must play a role in making institutions proactive and intentional in determining what data to collect, how to collect it, and how best to interpret and use it…” (7). Institutional research offices can develop effective partnerships and collaborations across the institution to facilitate data use. With an effective collaborative partnership, the institutional researcher and the content expert can discuss best approaches and help one another to better understand the data (4).
What might this look like?
To use an example in the context of performance-based funding and accountability:
Take, for example, the development of a state funding formula to determine distribution of state appropriations for public institutions. Both the institutional research professional and the representative from the department of higher education or the system office may benefit from candid conversations regarding the pros and cons about how legislation is drafted, the vagueness or appropriateness of goals, or how difficult it would be to answer a seemingly easy question that in reality is a very difficult question to answer…these relationships must be built on trust and respect for one another’s experience and expertise. (4)
I have had the opportunity to redesign the institutional research function at both community colleges and at a public regional university. Developing partnerships on campus is key, regardless of institutional positioning or size. As the institutional research executive, I have frequently attended faculty senate to hear the frustrations, obstacles, and opportunities encountered by the faculty. Similarly, I have attended department meetings across the organization, from finance to human resources to facilities, to understand the challenges that they face. By taking the time to understand the component units of the institution and to develop partnerships with them, my office was better able to develop effective data assets-including reporting, dashboards, and analytic visualizations-to address their business realities.
Access and literacy are key to the data-informed campus
If something cannot be measured and the resultant data stored, it is not possible to perform anything other than a “gut-feel” or purely qualitative assessment, a misstep that institutions cannot afford as enrollment and budget pressures increase. Investing in the professional development of faculty and staff to increase data literacy, as well as hiring data experts for the campus, will help the institution make more effective decisions and monitor the effects of various initiatives. For these professionals to maximize their returns, however, they must have access to adequate data resources and systems that will allow them to perform these analyses.
An effective institutional leader must be willing to make appropriate investments in both technological and human resources to support the data-informed revolution. To create a truly data-informed campus, the analytics organization’s infrastructure, staff, and duties must work in concert to facilitate better decisions and further the institution’s goals.
Sources (numbered throughout)
- Bichsel, J. (2012). Analytics in higher education: Benefits, barriers, progress, and recommendations. EDUCAUSE Center for Applied Research. http://www.educause.com/ecar
- Hillman, N., Tandberg, D., & Gross, J. (2014). Performance funding for higher education: Do financial incentives impact college completions? The Journal of Higher Education, 85(6), 826-857. https://doi.org/ 1080/00221546.2014.11777349
- Hora, M. T., Bouwma-Gearhart, J., & Park, H. J. (2017). Data driven decision-making in the era of accountability: Fostering faculty data cultures for learning. The Review of Higher Education, 40(3), 391-426. https://doi.org/ 1353/rhe.2017.0013
- Kirby, Y., & Floyd, N. (2016). Maximizing institutional research impact through building relationships and collaborating within the institution. New Directions for Institutional Research, 2016(166), 47-59. https://doi.org/10.1002/ir.20130
- McLendon, M., Hearn, J., & Deaton, R. (2006). Called to account: Analyzing the origins and spread of state performance-accountability policies for higher education. Educational Evaluation and Policy Analysis, 28(1), 1-24. https://doi.org/ 3102/01623737028001001
- Park, V., Daly, A. J., & Guerra, A. W. (2012). Strategic framing: How leaders craft the meaning of data use for equity and learning. Educational Policy, 27(4), 645-675. https://doi.org/10.1177/0895904811429295
- Peters, C. E., & Benitez, M. (2017). Leveraging a community participatory framework to move climate survey data into action at a small college. New Directions for Institutional Research, 2017(173), 63-74. https://doi.org/10.1002/ir.20213
- Spillane, J. P. (2012). Data in practice: conceptualizing the data-based decision-making phenomena. American Journal of Education, 118(2), 113-141. https://doi.org/10.1086/663283
- Taylor, J., & Machado, M. De Lourdes. (2006). Higher education leadership and management: From conflict to interdependence through strategic planning. Tertiary Education and Management, 12(2), 137-160. https://doi.org/10.1007/s11233-006-0003-3
More Blogs
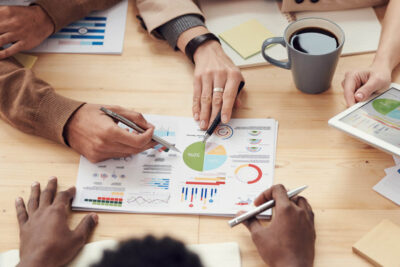
Why data governance matters for university strategy—and why most data governance projects fail
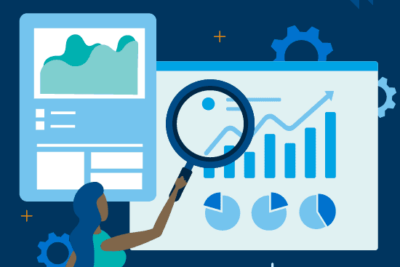
Is your data ready to support COVID-19 recovery?
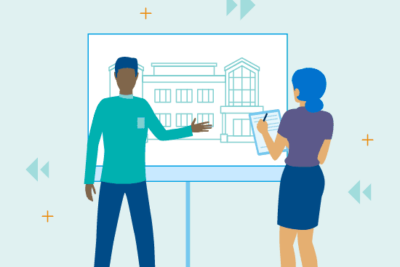