The promises and perils of student success predictive modeling
March 6, 2018, By Lars Waldo, Managing Director, Data Science
Prediction and ethics have always gone hand in hand. Go back to antiquity in literature or philosophy and you have endless stories about the promises and perils of prophecy. The reality today is that we’re getting much better at predicting the future. With big data, machine learning, and artificial intelligence, we don’t have to rely on crystal balls anymore.
But as predictive analytics become more ubiquitous at colleges and universities nationwide, the question on everyone’s minds seems to be: How do we make sure we’re using them right?
In this interview with Lars Waldo, we ask him for a data scientist’s take on the challenges and opportunities involved in predicting student success.
A quick primer on predictive modeling
When you hear “predictive modeling,” do you imagine a crystal ball? In reality, it’s closer to the weather app on your phone forecasting a 40% chance of rain on Thursday. Predictive modeling is the process of using statistical techniques to extract patterns from historical data in order to predict future outcomes.
At EAB, we take large amounts of historical data (10 years at some schools) and use a machine learning algorithm to transform the differences in student trajectories into an understanding of how different factors contribute to positive student outcomes. The algorithm looks for anything in the student data that correlates with those outcomes—like home state, first term GPA, the proportion of credits transferred in, or amount of unmet financial need. With every student record it looks at, the algorithm learns more about what leads to success and what leads to failure.
The result is a model that can look into a student’s past, against a constellation of historic data, and predict the likelihood of potential futures.
Q: What are the benefits of predictive models in student success?
A: The biggest benefit of predictive models is they can identify high-need students who are missed by traditional safety nets—and search entire universities for them in minutes. There are invisible patterns in student data that you or I might not be able to see, but that the computer can. It’s well known that it’s not just one thing that causes a student to drop out; it’s a complex matrix of factors that influences whether a student is successful. Through observation and experience, faculty and advisors have come to understand a number of risk indicators. But while some factors are easily-identifiable and make sense—like GPA—many of them are less intuitive.
A good predictive model can help us know which students might need help even when they aren’t tripping anyone’s internal alarm bells. And those factors change for different types of students and at different points in the student lifecycle. We’ve found instances where factors are things like the variance in a student’s grades or even their trend in attempted credits over time.
Predictive models can also help us deal with our capacity issues. Great advisors know specific things to look for in the data and can talk to a student and uncover potential risk factors that a computer would never find. But it will take a lot more time. And you can imagine doing that for every single student in an advisor’s caseload is just impossible. For the most part, this means that institutions never have a comprehensive picture of risk across their entire student population. A predictive model can give a complete risk assessment for an entire institution much faster, making it so that faculty and staff can prioritize and tailor their efforts.
Q: What is the hardest thing about trying to model student outcomes?
A: We are trying to model a very complicated process and we know that the data can never give us a complete picture of what’s going on in the life of a student. The series of decisions, over time, that eventually lead a student to drop out of school involves important human factors that just can’t be measured and put into a database, so we know that we’re trying to build a model without the whole picture. In some cases we can use proxies to measure such factors—for example, parents’ method of payment can be very telling about whether a student is facing financial difficulty.
In other domains, the factors that ultimately contribute to the outcome are more readily collected. This is particularly true in domains that involve small transactional decisions rather than a series of large potentially life-changing decisions. For instance, Uber uses traffic and weather data to predict your trip duration, and Amazon uses predictive models to stock a portfolio of products for quick delivery; neither of these processes involve a lot of unobservable data about human behavior. Modeling student success is more like modeling patient outcomes in healthcare. We are trying to model a complex socio-behavioral process, and while our predictive model is vastly superior to every intuition we may have, it is still far from the proverbial crystal ball.
Q: People talk about the “perils” of predictive modeling. What do they mean?
A: I think this is a very important topic and will become more important as we make progress in artificial intelligence and predictive modeling. As engineers, we aim to design the most accurate models. This is a noble pursuit, but we have to be very mindful about how the models will be used or potentially misused. Models don’t predict the future—they describe the past and we are the ones making the “prediction” that the future will continue to work in roughly the same way. This means that they have the potential to reinforce historical patterns, including ones we would rather do away with. In student success, risk assessment based on race or socioeconomic status is controversial. Our biggest concern is that predictive models paint a discouraging picture of a student for reasons outside of their control, and that it ultimately has a negative impact on the kind of support he or she receives.
Predictive models do not have a moral compass, so I believe the burden is on the shoulders of data scientists and engineers to design these systems with open eyes. We need to work closely with domain experts and researchers to make sure that we design for the right objective. Good decision support tools are derived from research and built with a use-case in mind. Models are not useful if they are just making predictions for prediction’s sake. Models are useful if their insights lead to actions that change outcomes for the better. If we are not designing a model with this mindset, we are not doing our jobs.
Q: What is one thing you wish you could tell members about student success modeling?
A: I would tell them to fight the natural craving for certainty when thinking about the model. It is very easy to overestimate the probability of likely events, or underestimate the probability of unlikely ones. If the model says 1,000 students have a 10% chance of graduating, then 100 of them will probably graduate. This seems obvious when stated that way and yet each of those hundred students would probably surprise those around them especially if they knew that student only had a 10% chance. Predictive model scores are not fate; they are an empirical estimate of likelihood. Unlikely things happen all the time, so embrace the uncertainty and remember that you still have power over the final result.
Q: What do you see as the future of student success predictive modeling? Where is it headed?
A: I think we are already in the middle of a major shift toward more student-centric data. A few years ago, we all started our journey with “frozen” data—things that are set in stone about a student, like their hometown, ethnicity, age. Most of the original retention and graduation studies were based off of this kind of data, and it provided a great foundation, but it misses a huge chunk of what is really going on in students’ lives.
We’ve been continuously moving toward new data sources that can give us different signals, particularly about student engagement because it’s the hardest to observe in traditional student data. We’re looking at real-time, granular data streams that show the tiny interactions and transactions that students are having with the institution on a daily basis. That’s why you’re seeing increasing interest in things like service appointment data and learning management system data. We are also excited about the data we’re now collecting through the student-facing elements of our EAB products. I think the culmination of these new behavioral data sources will allow us not only to make better models but to also make models focused on assessing different dimensions of risk. That would be a major step towards triggering interventions, which in my mind is the next wave of innovation for us.
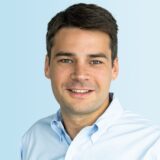
More Blogs
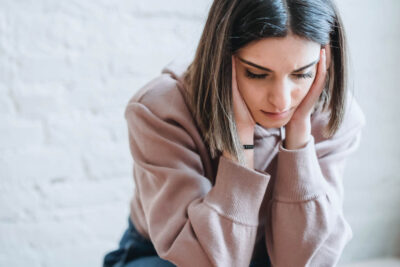
3 ways to combat the student loneliness epidemic on your community college campus
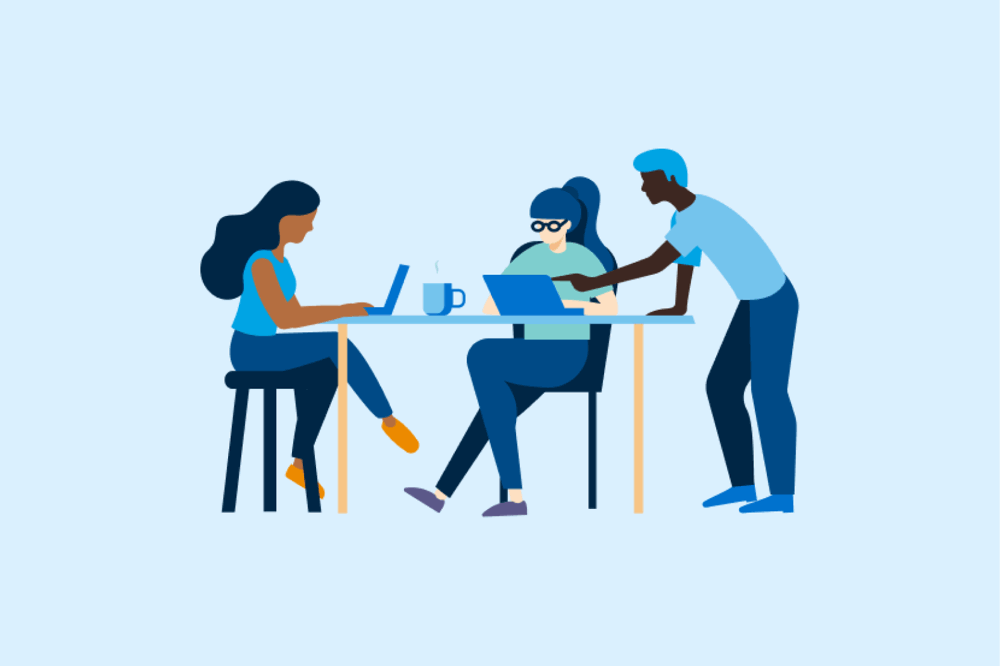
Students need career support earlier. Here’s a scalable way colleges can provide it.
Student Success Blog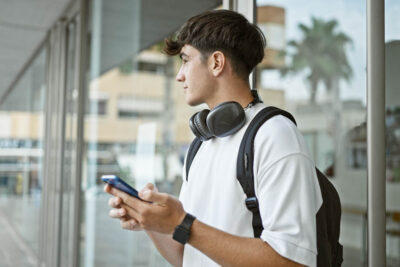