How to Solve the Data Activation Challenge at Your Institution
Episode 117
August 23, 2022 • 21 minutes
Summary
EAB’s Earl Sires and Mike Laracy examine the “digital sprawl” problem that plagues many college campuses and discuss tips and tools for cutting through all that complexity. Rather than relying on overworked programmers and statisticians, the two urge campus leaders to use an education data platform to tame the sprawl and facilitate direct access to the data by those tasked with making strategic decisions.
They also demystify terms like predictive modeling and identify use cases that illustrate how critical advanced data analytics can be to establishing a more effective, data-informed culture at your school.
Transcript
[music]
0:00:13.0 Speaker 1: Hello, and welcome to office hours with EAB today’s episode is focused on a problem that plagues the vast majority of college campuses, namely digital sprawl, our experts offer a brief tutorial on how to tame, access, and extract useful insights from the digital rats nest that your IT folks probably refer to as a data ecosystem. Don’t worry. The episode is short and we’ve stripped away as much tech jargon as we possibly can. So give these folks a listen and enjoy.
[music]
0:00:47.3 Earl Sires: Hello and welcome to office hours with EAB. My name is Earl Sires, and this is my first venture into the world of podcasting, but I’ve been studying the problem we’re going to be talking about today for some time, that topic is the way that higher ed institutions use or fail to use in some cases, data to understand what they’re doing right versus what they could be doing better. Joining me today is my colleague, Mike Laracy. Mike, would you mind introducing yourself and telling us what you hope listeners will take away from our conversation today?
0:01:20.7 Mike Laracy: Yeah sure. Thanks Earl. And this is my first podcast as well. So I’m a managing director here at EAB. I founded a company called Rapid Insight where we developed tools to help organizations extract data… Extract value from their data and build predictive models. Last October, Rapid Insight became part of EAB and I’m continuing to work on Rapid Insight’s mission now as part of the EAB team as far as what I hope listeners take away from this. I hope that we can provide a couple of tidbits of information on increasing organizational intelligence.
0:02:06.3 ES: Okay. And that phrase, organizational intelligence that you use, could you expand on that a little bit?
0:02:12.4 ML: Yeah, it’s pretty much the same thing as human intelligence, but for an organization. So if you think about a human being who’s really good at retaining and storing information and also really good at recalling and processing that information to make decision that… We would call that a smart person. So organizations you can think of in the same way an organization stores and retains information in the form of data, it recalls and processes that data in the form of data analytics. So an organization that can do this well is a smart organization and an organization could be anything. It can be a college, a business, a hospital, whatever.
0:03:00.2 ES: That’s a pretty interesting way to think about it, where data and analytics are kind of the brains of the organization and using that data intelligently is, well, like you said, intelligent. So with all that in mind, I was just curious if you could talk a little bit about the current state of affairs at most institutions, just to help set the context for why this issue is so important.
0:03:20.2 ML: Yeah, definitely. So I’ll focus this on higher ed, obviously. So colleges and universities are a wash in data. They’ve got tons of data in all different systems. But they, a lot of colleges and universities really lack the staff capacity to be able to take that data and turn it into actionable information. And most data is siloed, it’s stored in different places like CRM systems, student information systems, you name it, all kinds of systems. So that data is kind of difficult to get to, difficult to analyze, difficult to extract insights from. So as in the case with most industries, there’s a lot of room for improvement here.
0:04:12.0 ES: Okay. So like you said, data often, it is stored in different departments. They have their own protocols and practices for storing and accessing that data and they can’t necessarily be accessed by other departments, or it’s siloed in some other fashion. So I was just curious what you think might be an efficient way to solve that problem without having to blow up your entire IT infrastructure and start over from scratch since that’s definitely not a practical solution for most institutions.
0:04:37.6 ML: Yeah. You definitely don’t want to start over from scratch. So given all the data that lives in all of these systems, if an institution does not have a data warehouse, that’s definitely a good place to start. Here at EAB, we’ve got a great data warehouse called Edify that kind of ingest and organizes the data from all these systems puts them in one place and allows people to access that data from the data warehouse. But that solves kind of half the problem. The other half of the problem is that you really need people to analyze this data, to be able to get to it, to be able to turn it into actionable insight. And gone are the days where you can rely on teams of SQL coders or large teams of data scientists really you need a lot of people to be able to get at this data and to build predictive models, report on outcomes, run analysis, answer an ad hoc question that kind of thing.
0:05:50.8 ES: Okay. So you mentioned predictive modeling in particular, and it sounds before you know much about it, like sort of an esoteric topic that you really need to know quite a bit about math to understand. So I know from a few years of working with you and learning from our partners, that it’s not quite as complicated as it seems in terms of how it’s applied. It’s definitely, at its core, a lot of math that goes into predictive modeling, but I was just curious if you could explain for the layperson what you mean when you talk about predictive analytics and predictive modeling. And if you could also give an example or two to help people kind of wrap their heads around the concept and how it is actually manifested.
0:06:26.1 ML: Yeah, sure. So, yeah, it sounds a little scary but it’s really not, it’s less complex than people think it is. So predictive models are mathematical equations that use data to make predictions about things. So in higher ed, predictive models are used for things like, predicting enrollment probability for applicants, or attrition probability for students, or summer melt likelihood for incoming students. And outside of higher ed, some examples, supermarket chains use predictive modeling to determine price elasticities, and figure out what the optimal price for a product would be. Credit card companies use it to predict when fraud is occurring. Hospitals use it to predict patient risk. So it can be used for anything across any industry, and really, it’s just equations that use historical data to predict things in the future.
0:07:32.5 ES: So it sounds like there’s actually a lot of room for creativity and flexibility in the sorts of things that you can apply predictive modeling to.
0:07:39.3 ML: Definitely. Yeah. There’s all kinds of things that can be done with predictive modeling, and data analytics in general.
0:07:47.3 ES: And what percentage of colleges and universities would you guess are using data and predictive modeling at the level of sophistication that you’re talking about with these enrollment models, summer melt models, all the different types of models that you might build? What percentage would you say are using it to the capacity that they could be, and what are some of the common reasons that institutions aren’t using it as well as they could be?
0:08:09.8 ML: Yeah. So as far as what percentage are using it to its full capacity, I don’t really have a good answer for that, because I would say every school, every college and university is using analytics in some form, but it’s all at varying levels. So there’s certainly a percentage that do it very well, there’s a percentage that do it average, and a percentage that do it poorly. But it’s really hard to say the exact percentage of institutions that are doing it, doing it well.
0:08:47.6 ES: Well, over the years that I’ve worked with you and our partners in higher ed, I’ve heard sort of a shift in the language that partners use to describe the way they’re working with data. And it’s seems like a distinction that’s really kind of cropped up recently. A lot of schools talk about becoming data-driven. That’s sort of the aspiration that they’re working towards, looking to the data to make calls about how to optimize their operations, and increase enrollment, and all those different objectives, and performance goals that they have. But I think over the past few years, people have spoken more about becoming data-informed rather than data-driven. So looking at data more as a piece of a larger picture, kind of one piece of the puzzle, and it takes a human to make judgment calls and assemble that puzzle. So an example of data-informed decision-making that comes to mind for me that a partner developed recently, was a revenue attribution dashboard that they built, and it really allowed them to drill into their data and see which different sections and majors and faculty brought in revenue for the institution, and which of those different elements operated at a cost.
0:09:54.7 ML: Very cool.
0:09:55.5 ES: I remember… Yeah. I remember that partner telling us about how, rather than just looking at that data and saying, “Okay, we’re going to cut or consolidate everything that doesn’t contribute revenue,” the school looked at it in a more nuanced way through the lens of their larger mission, and their objectives as an institution. So if they had separate programs for the organ and the piano, just as an example, maybe the piano contributed revenue, but the organ didn’t contribute revenue. So instead of saying, “Let’s combine these into one track and consolidate them since only one of them is producing revenue for our school,” they decided that having a really large and diverse set of instruments in the orchestra was really important to their institution’s identity, so they decided to keep both of those instrument tracks and then make a consolidate… Consolidation decision somewhere else, somewhere that wasn’t quite in support of their institution’s mission. So that was kind of an example that came to mind of making data-informed decisions, rather than purely data-driven decisions. And I was just curious what you thought of that distinction, and how that tends to play out at higher ed.
0:10:56.8 ML: Yeah, so, I don’t know, for me, it’s really, that’s a change in terminology, but they mean the same thing. So you don’t really want data driving your decision. You want data to inform your decision. So it’s not like you point at the data and it gives you the answers. It’s, you look at the data, and then human beings need to be involved to interpret that data. So you want the data to inform your decisions, but if the data’s driving your decisions, that it makes it sound like you’re just relying on the data to tell you what to do, whereas you… Interpretation needs to happen of what the data is actually saying.
0:11:36.5 ES: And bringing some of that judgment and experience in human evaluation into the equation, when we’re looking at some of the specific pain points the schools are facing right now, it seems like staffing is one that keeps coming to the surface as one of the most prominent issues that institutions are dealing with, especially in the more technical roles like institutional research, or in IT. So I was wondering what role data and analytics technology might play in easing that crunch that institutions are dealing with at the moment.
0:12:09.8 ML: Yeah. So staffing is an issue in every industry these days with The Great Resignation. But for in the world of data, it’s been a real issue for, I would say, at least a decade now. There’s just not enough data scientists, not enough programmers to do the work that’s needed. So really, I think the solution to that is, not relying solely on programmers and statisticians, and relying more on people that can analyze the data themselves. So yeah, I think that is the only thing that can help the staffing issues in the world of data and analytics is more people need to be able to do it, so we need to create those analysts as opposed to find them, if that makes any sense.
0:13:15.4 ES: Yeah, it does. And I think at times when we talk about that concept with partners of self-service analytics, which is sort of how you described it earlier, putting data in the hands of staff who might not ordinarily be thought of as staff members who would be functionally data analysts, sometimes schools have a really positive reaction to that, and they immediately think this is what we need to do and where we need to go. And then at other times, schools have a little bit more of a reluctant reaction, because they wonder about releasing data for anybody to view, sometimes that could create trust issues or privacy concerns. So I was just kind of curious for schools who do have a more reluctant take on self-service analytics, how would you address some of those concerns?
0:14:00.2 ML: Yeah, so if you look at the two extreme views, I don’t know if anybody has these views but we just assume that on one side it is lock all the data up and don’t let anyone access it, and on the other extreme it’s just let anyone access the data that wants to access it. And I think both of those, they’re the extreme, so neither of those really make sense. I think the answer is somewhere in the middle, where you need to be able to have policies in place of who can access the data and when, and why. Good data governance policies and things like that. But you really want… If an institution wants to become smarter, they absolutely need to provide access to the data. They can’t just limit the data access to a small number of IT staff or things like that. If they do that, there’s no way to answer all of the questions that can be answered from the data. So hopefully that makes sense.
0:15:12.9 ES: It does. Would you…
0:15:16.1 ML: Sorry. Earl and I have a good history of interrupting each other at the same exact time. [chuckle] So I think that kind of goes back to the need for a data warehouse, and one important aspect of that is data lineage. I don’t know, Earl, you’re probably familiar with that term. I don’t know if you want me to explain that or not.
0:15:42.0 ES: Yeah, that might be good. Just to clarify a little bit about what data lineage is.
0:15:46.2 ML: Yeah. So data lineage is essentially a map of the data itself. So if you’ve got all this data coming from multiple different systems, you’ve got things that are happening to the data, it’s being transformed, it’s being aggregated, and if you don’t have… If somebody doesn’t have the knowledge of where the data came from and the journey that the data has and data had been on to get there, it’s very difficult to be able to analyze that data. So data lineage is, like I said, a map of where the data came from and what happened to that data along the way.
0:16:26.9 ES: And does that play into the concept of the data dictionary at all?
0:16:34.0 ML: Yes. Yes. The data dictionary will explain usually the fields and the variables, but it’s not always… It doesn’t always give you the data lineage. So, data dictionaries are necessary, and sometimes the data lineage is contained in those data dictionaries, but really both are needed.
0:17:00.4 ES: And it’s definitely true that a lot of partners of ours, but also institutions in general, are working to build data warehouses. And I think it’s just become recognized as a necessity for a lot of schools that have all of these different systems. Student information systems, learning management systems. A lot of times that data is stored in different ways on all those systems, so people can’t really get a comprehensive picture of what’s going on for individual students when not all that information is easily available. So I was curious if you had any thoughts on data warehousing and maybe some other important considerations or objectives that schools should be looking toward when they’re building data warehouses.
0:17:37.9 ML: Yeah. So I would say if you don’t have a data warehouse it’s fairly integral for increasing your organizational intelligence. Regardless though of whether or not you have a warehouse, you need to work to create a data informed culture, ’cause people don’t know what they don’t know. So if people within the organization don’t know that they can ask questions of the data, they’re not gonna ask them. So it’s really important to work, and it’s not easy, it’s a lot of work is involved, but you need to create that data-informed culture. And if you’re starting from scratch, the best way to do this is by starting out small, hit a couple of single… Don’t go for the home run right away, maybe pick a couple of small analyses that will answer the questions, a couple of questions that are needed for your organization. And once people see that the data can tell you, the data can provide you with information that you need, they’ll get onboard. Because the more people that see that data can inform decisions, the more they’re gonna want to ask questions on that data.
0:19:02.8 ML: So if you don’t have a warehouse yet, start out by at least creating a data dictionary and defining where all the data is and what the data is, and get access to tools that will allow people to answer those questions quickly. Rapid Insight, in my biased opinion, is a good tool for that. Edify is an incredible warehouse. And these two technologies combined can help increase your organizational intelligence.
0:19:39.6 ES: All right, well, thanks a lot, Mike, and I think since we’re talking about a pretty complex subject and our time’s a little bit limited, but it was great to get those concrete takeaways and steps from you that institutions can use to reflect and make sure they’re moving in the right direction. So I just wanna say thanks a lot, Mike, for your time today. And I really encourage our listeners to check out the Edify and Rapid Insight links that are included in the show notes. And until next time, thank you for listening to Office Hours with EAB.
0:20:11.2 ML: Thank you.
[music]
0:20:18.4 S1: Thank you for listening. Please join us next week when we dig into the findings of our latest survey of parents and guardians, to find out what role, if any, they have in their sons’ or daughters’ college search these days. You just might be surprised by what we found. Until next week, thank you for your time.
More Podcasts
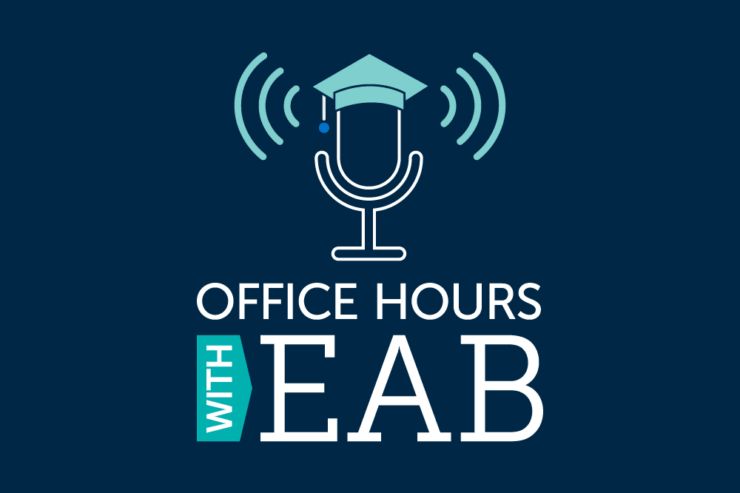