Creating a data-informed campus: part 1
3 requirements for your tech infrastructure
September 21, 2022
Glancing at any higher education publication or public discourse, it’s easy to see that higher education is operating in a period of enhanced scrutiny. Accreditors, state legislatures, and the general public are calling for increased accountability. The “completion agenda” focuses on increasing retention and graduation rates on campus. A focus on workforce preparation asks colleges to justify investments in programs based on return on investment and student placement rates. The need for information continues to increase. Fortunately, data and analytics can help institutions of higher learning to address these data needs.
In my role as both a consultant and an institutional effectiveness leader, I have witnessed many challenges that hinder the creation of a data-informed campus. Many institutions rely on aging operational systems, fail to staff an analytics function appropriately, or face resistance on campus to the use of data to drive decisions. A successful analytics function requires a functioning technology infrastructure and both financial and operational support.
This blog post is the first in a series examining how you can create a data-informed campus. I’ll discuss three necessary elements of technology infrastructure: data integration, data governance, and business intelligence. In future blog posts, we will look at staffing the analytics function and explore the key roles of the emerging analytics discipline in higher education.
3 elements of technology infrastructure you need for a data-informed campus
1. Data integration
A data system that provides easy access to reliable data from campus systems is the backbone of a data-informed campus. Many systems that exist on a campus are aged enterprise systems that were not designed to meet the analytic and strategic reporting needs of today’s higher education environment. As one report noted, “Maintaining a sound infrastructure can help ensure that cleaning, sharing, and using large amounts of data for making decisions institution-wide can be carried out smoothly and that different data systems can ‘speak’ to one another.”
As information is gathered from various sources, this information can be stored in a data warehouse. For example, a data warehouse can extract information from the student information system and the student success management system. This information can then be normalized and stored in a structure that is better suited to reporting and business intelligence initiatives.
As data is loaded into a warehouse, transformations can be applied to increase the value of the data. For example, as data is ingested the warehouse might look at data from the student’s admission application or federal financial aid submission to determine the student’s status as a first-generation student. By incorporating this logic into the data extraction and warehousing process, the same logic and results are shared by all users of the data warehouse. This increases the consistency of data across campus. An outcome of the data ingestion process and logical processes is a semantic layer, which is a centralized and curated source of data for analytics and reporting initiatives. This semantic layer enhances the reliability of the data and also helps to inform data governance efforts on the campus.
Modern Infrastructure In Action: Explore the Case Studies
2. Data governance
One of the most frequently overheard buzzwords in the higher education discourse is “data governance.” This abstract concept takes many different forms; however, at a high level, data governance incorporates organizational guidelines to ensure data quality and accountability. In 2018, scholars Jim and Chang created a data governance checklist that incorporates eight major criteria derived from key literature:
- Data governance body or stakeholder group
- Data quality
- Data access or restriction
- Data security
- Data stewardship
- Ownership or roles
- Metadata documentation or organization
- Business process integration
In terms of your technology infrastructure, data warehouses and data dictionaries can help to facilitate data governance. For example, EAB’s Edify includes the ability to define any element in the data model and to comprehensively document data ingestion and transformation processes.
In addition to technology, however, there must also be a significant institutional commitment to governance at three levels: executive, strategic, and operational. Setting the overall campus strategy and vision for data analytics is an executive-level function. Strategic-level discussions may focus on assessing various data sources, establishing data definitions, or considering the overall data access posture of the institution. Finally, operational data governance may focus on more tactical matters, such as defining project scope, plan, and results.
Data governance touches all areas of a campus; an external tool can be useful in creating an overall governance strategy. To facilitate a data-driven environment, the institution must have a well-designed analytics solution that combines data from a variety of disparate sources in a singular, governed environment. Extracting and integrating data from various systems across campus into a central data warehouse is a first step, but this must be combined with a robust data governance regimen to an effective institutional analytics function.
3. Business intelligence
Access to and use of data are potential limitations to an effective data-driven environment. Business intelligence solutions are designed to facilitate easier access to data for reporting and analysis. Business intelligence tools can provide an avenue for organizational constituents to access and interact with data. This interaction has been made easier by the addition of data warehousing on many campuses, which reorganizes and integrates data to facilitate analysis.
Data visualization tools have emerged as a primary solution for business intelligence delivery. These tools provide a structured environment where end users can explore data. This may occur through the development of dashboards by a centralized institutional research or effectiveness function, by allowing end users to access a data within a curated visualization tool (commonly referred to as “self-service analytics,” or by some combination of these two approaches).
When designing visualization solutions, the needs of the end user should always be paramount. For example, Aguilar, Lonn, and Teasley’s study of early-alert warning systems found advisors were using dashboards during advising sessions. The original dashboard was designed to identify students who were experiencing academic struggles; using it during the actual advising session was an unintended use case that required a redesign to enhance effectiveness. For use with students, the dashboard was redesigned to ensure the peer names were not visible and graphics were more informative. When designed with the user in mind, dashboards and visualizations are an excellent entry point to working with institutional data across a number of constituent groups. Executive leadership, Boards of trustees, and other high-level constituencies can access summary dashboards created by a central institutional research or effectiveness function, while individual departments can employ curated datasets to address specific inquiries.
Infrastructure improvements are a necessary first step
The need for easily accessible, relevant, and actionable data continues to grow as calls for accountability increase, and campuses try to strategically employ limited resources. Campuses must address technology infrastructure as they move toward becoming more data-informed, and necessary technological components include a data warehouse coupled with data governance policies and practices. In addition, a robust business intelligence solution helps to free the data and make it more easily accessible to both decision-makers and interested parties. In upcoming blog posts, we will examine how to optimally staff the burgeoning analytics function, as well as explore how data might be employed in practice.
Sources (linked throughout)
- Ekowo, M., & Palmer, I. (2017, March). Predictive analytics in higher education: Five guiding principles for ethical use. https://na-production.s3.amazonaws.com/documents/Predictive-Analytics-GuidingPractices.pdf
- Jim, C. K., & Chang, H. (2018). The current state of data governance in higher education. In L. Freund (Ed.), Proceedings of the Association for Information Science and Technology (pp. 198-206). Wiley. https://doi.org/10.1002/pra2.2018.14505501022
- Friedrich, F. (2013, December 6). Good BI governance is just good business. EDUCAUSE Review. https://er.educause.edu/articles/2013/12/good-bi-governance-is-just-good-business
- Kerrigan, M. R. (2014). A framework for understanding community colleges’ organizational capacity for data use: a convergent parallel mixed-methods study. Journal of Mixed Methods Research, 8(4), 341-362. https://doi.org/ 1177/1558689814523518
- Drake, B. M., & Walz, A. (2018). Evolving business intelligence and data analytics in higher education. New Directions for Institutional Research, 178, 39-52. https://doi.org/10.1002/ir.20266
- Aguilar, S., Lonn, S., & Teasley, S. D. (2014). Perceptions and use of an early warning system during a higher education transition program. In Proceedings of the Fourth International Conference on Learning Analytics & Knowledge, ACM (pp. 113-117). https://doi.org/10.1145/2567574.2567625
More Blogs
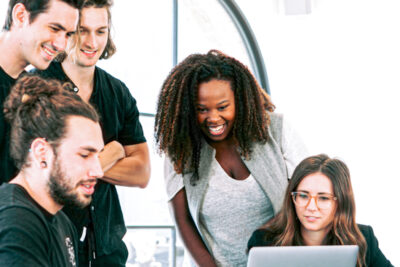
3 reasons your colleagues don’t want to use data—and how to change their minds
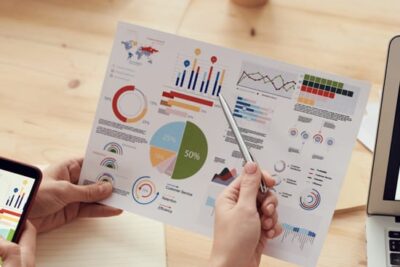
Where are the gaps in your data strategy?
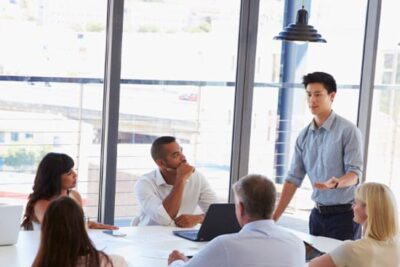