Creating a data-informed campus: part 2
How to staff your analytics function
November 9, 2022
The need for data-informed decision making in higher education continues to increase. As I discussed in the first blog post in this series, institutions need a sufficient technology infrastructure to facilitate curation, access, and retrieval of data. But successful adoption of new technologies requires more than just the technology itself—it also requires the necessary leadership to set a clear vision for analytics and data in the organization, and sufficiently qualified staff to execute upon this vision. In this post, I’ll detail the structure and staff needed for a modern analytics function.
“Learning professionals would be well-served to consider the kinds of decisions that data analyses will be likely to serve and not put their faith in the misguided belief that big data will serve up ready-to-go solutions from the mist” (1).
The optimal structure for an analytics function
Higher education leaders often overestimate the analytics, data, and information capabilities of the institution (2). In reality, education lags behind all other sectors in adoption of analytics solutions (3). With the need for a supportive organizational infrastructure in mind, traditional reporting structures may no longer work as desired. The 2019 Campus Computing Project survey of chief information officers and senior campus officials found the following (4):
The 2019 Campus Computing Project found the following (4):
43%
Of campuses had reorganized information technology within the past 2 years55%
Had reorganized in the past 2 years and planned to do so again in the next 2 years45%
Anticipated an information technology reorganization within the next 2 yearsFor analytics initiatives to achieve maximum effectiveness, the analytics function must be visible and connected within the organization. The optimal structure is a centralized, integrated analytics function reporting to senior institutional leadership helps to reduce the perception of internal politics and signal the strategic priority of the function (5).
“Analytics programs are most successful when various constituents – institutional research, information technology, functional leaders, and executives – work in partnership” (6).
Leimer introduced the concept of the integrated model, an office that incorporates traditionally disparate offices that provide data and decision support – institutional research, assessment, strategic planning, accreditation, and other operational units – into one office dedicated to data-driven decision making and leadership. The concept of an integrated model is partially responsible for the increase in institutional effectiveness offices. While institutional effectiveness is sometimes a rebranding of institutional research, in the integrated model, institutional effectiveness is “an umbrella title for a unit or department that performs multiple ‘quality functions'” (7).
When I arrived on the Utah Tech University campus as the inaugural executive director of institutional effectiveness, I inventoried the disparate functions that contributed to data and decision support. The campus had a strong compliance-oriented institutional research function, but other functions such as strategic planning and accreditation did not have a formal home. Considering the integrated model (proposed by Leimer) along with successful models at other institutions, I began architecting the institutional effectiveness function by assessing the organizational structure.
Waht IT & IR Collaboration Means for Campus Analytics
Indeed, as you move toward a data-informed campus, assessing your organizational structure is necessary to ensure success. Rehoming disparate units such as institutional research (the traditional compliance reporting unit), business intelligence functions from information technology, accreditation, assessment, and strategic planning into one cohesive function helps to closely relate data, reporting, and analytics activities with the needs, mission, and vision of the institution. The consolidated office may also offer staffing efficiencies and incremental gains from knowledge transfer as individuals from previously disparate units share their expertise.
4 roles you need in your analytics function
Creating a successful and effective analytics function requires a diverse skillset, and often necessitates skills that historically may not have existed in higher education. The diversity of skills needed in the analytics function creates new employment opportunities, but also presents commensurate challenges. While staffing will depend on the structure and role of the organization, necessary skills will continue to evolve. In an ideal analytics organization, executive, functional, and technical roles are necessary to support the organizational mission.
1. Executive leadership
Sufficient executive authority and strategic leadership is integral to success. The analytics function should be headed by an executive-level administrator who can bridge the gap between academic and institutional priorities and the technical workings of the analytics function. The leader must be a strategic thinker and possess the ability to examine the whole institution, prioritize data analytics needs, design appropriate data analytics, reporting, and visualization solutions, and guide and motivate the analytics staff. This role exists in various forms at present, often splitting roles between a director of institutional research, chief information technology, or director of institutional effectiveness (7).
The institutional effectiveness function continues to evolve and often oversees the integrated model. When I took my role at Utah Tech University, I worked closely with the President and the executive team to situate the executive director role at the appropriate level of influence within the organization, reporting directly to the President. This allowed me to maintain an awareness of the strategic priorities—as well as tangible and perceived threats—facing the institution, and to develop data and decision-making solutions that allowed the institution to more quickly respond.
Watch the Webinar: Leadership Perspectives on Campus Data Strategy
2. ETL specialists
Data extraction-transformation-load (ETL) specialists or data warehousing specialists focus on programming, data integration, and data warehousing (8). These individuals work primarily in structured query language (SQL) and other specialized tools to connect and retrieve data from disparate campus systems. This role is almost purely technical in nature, although domain knowledge can help the analyst to better understand the various data sources. These individuals help ensure that data is in a format that is appropriate and accessible for various reporting and analytics tools.
3. Data analysts
A role that exists in most institutional research offices today, the data analyst role focuses on retrieving and analyzing datasets from the data warehouse and other campus data resources. A deep knowledge of database management, statistical analysis, and research techniques are generally necessary skills for an analytics team. While SQL has been in use to retrieve data from relational databases since the 1970s, new business intelligence tools such as Python, R, and Tableau for managing, extracting, and visualizing data are rapidly being employed in the academy (9). The more specialized tools the analyst is familiar with, the more he or she can apply versatile methods to work with complex data. Analysts may also specialize in particular tools. For example, some institutions have data visualization analysts or business intelligence analysts, dedicated solely to creating dashboards or other information reporting.
Employing individuals who can understand the data is critical. This understanding encompasses both the technical structure of the enterprise data system and how to query and retrieve necessary data, as well as the broader implications of the data retriever. The data analyst role, therefore, must have sufficient domain knowledge and develop institutional relationships. Helping data requestors and executive leadership to understand the data and to iterate appropriate visualization solutions markedly increases the effectiveness of the analytics function.
In larger institutions, a functional analyst role may be appropriate. This role works with “power users” in the organization who may create data visualizations or reporting based on a prescribed semantic layer or curated dataset provided by the analytics function. Functional analysts can work with constituents across campus to teach them how to conduct analysis or glean insights from data.
4. Data scientists
The data scientist is generally perceived as a rare amalgamation of the individual analyst roles (8). These individuals incorporate the skills of several other roles, by “merging the skills of statistics, mathematics, programming, communication, and business knowledge” (8). The data scientist may conduct more sophisticated institutional analysis, using machine learning theories such as sentiment analysis or network analysis.
-
78%
Of respondents report that it is increasingly difficult to hire information technology talent due to off-campus competition and salaries (4)
Hiring qualified staff with sufficient knowledge and expertise in data and analytics is a major challenge facing colleges and universities (6). Institutions may need to examine their talent acquisition and development practices, including increasing financial compensation to appeal to new hires (5). Analytics talent can often command higher salaries and other benefits such as bonuses and stock options in the private sector.
Using your data requires the right people
As I discussed in the first blog post of this series, technology infrastructure is certainly an important component of creating a data-informed campus. However, the appropriate staff and organizational framework to execute on data and analytics initiatives is equally important. Even the best technologies can languish without organizational commitment and employees that can help strategize, focus, and facilitate the development of the data-informed campus. In the upcoming and final blog post, we will explore how data might be employed in practice to facilitate institutional effectiveness.
Sources (numbered throughout)
- Wagner, E. (2012). Eight realities learning professionals need to know about analytics. T+D, 66(8), 54-58.
- Norris, D. M., & Baer, L. L. (2013). Building organizational capacity for analytics. EDUCAUSE. https://library.educause.edu/-/media/files/library/2013/2/pub9012-pdf.pdf
- Macfadyen, L. P., Dawson, S., Pardo, A., & Gašević, D. (2014). Embracing big data in complex educational systems: The learning analytics imperative and the policy challenge. Research & Practice in Assessment, 9, 17-28.
- Green, K. C. (2019). Campus computing 2019: The 30th national survey of computing and information technology in American higher education. https://www.campuscomputing.net/content/2019/10/15/the-2019-campus-computing-survey
- Krawitz, M., Law, J., & Litman, S. (2018). How higher-education institutions can transform themselves using advanced analytics. McKinsey Insights. https://www.mckinsey.com/industries/social-sector/our-insights/how-higher-education-institutions-can-transform-themselves-using-advanced-analytics
- Bichsel, J. (2012). Analytics in higher education: Benefits, barriers, progress, and recommendations. EDUCAUSE Center for Applied Research. http://www.educause.com/ecar
- Leimer, C. (2012). Organizing for evidence-based decision making and improvement. Change, 44(4), 45-51. https://doi.org/ 1080/00091383.2012.691865
- Wishon, G., & Rose, J. (2016). Institutional analytics and the data tsunami. EDUCAUSE Review. https://er.educause.edu/articles/2016/12/institutional-analytics-and-the-data-tsunami
- Drake, B. M., & Walz, A. (2018). Evolving business intelligence and data analytics in higher education. New Directions for Institutional Research, 178, 39-52. https://doi.org/ 1002/ir.20266
More Blogs
Improve your IT talent pool with candidate-centric recruiting
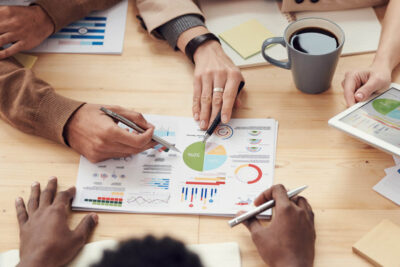
Why data governance matters for university strategy—and why most data governance projects fail
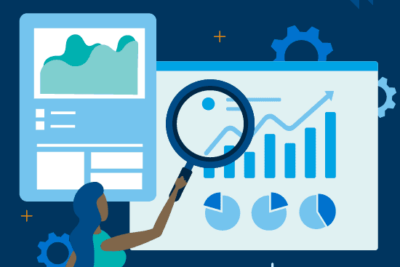