Building a comprehensive prospect-to-applicant model
Integrating demographic, academic, and engagement metrics for enhanced enrollment forecasting
December 14, 2023
Jennifer Lee
Assistant Vice President for Enrollment Systems, University of North Texas
The views and opinions expressed are those of the author and do not necessarily represent the views or opinions of EAB.Â
Topic
In the dynamic and competitive landscape of higher education, initiative-taking institutions are consistently seeking out and adopting innovative methods to optimize the student recruitment process. A comprehensive understanding of the likelihood of a prospective student’s decision to apply is not just advantageous—it is crucial. It enables institutions like ours to judiciously allocate resources, devise targeted marketing tactics, and refine overall recruitment endeavors.
Our current prospect-to-applicant scoring model, integrated within our Customer Relationship Management (CRM) system, serves as a strategic tool in our efforts to predict application probabilities and to tailor our engagement strategies accordingly. While the model is informed by a range of inputs and historical patterns, we recognize the importance of continuously enhancing its predictive power.
-
Interested in the Rising Higher Education Leaders Fellowship?
Learn more about the program and future cohorts.
Get Started
Machine learning solution
Machine learning models present a robust solution, capable of sifting through vast amounts of data—from student interactions with emails to campus event attendance—to predict application likelihoods with greater accuracy.
For the project, I explored various machine-learning models. The K-Nearest Neighbor (KNN) model emerged as the front-runner, owing to its high degree of accuracy. KNN operates on the principle that similar things exist nearby. It predicts a student’s likelihood of application based on the similarity to past applicants. This type of model is particularly useful in identifying nuanced patterns in the data, which can be crucial for determining the strategic allocation of our recruitment efforts and resources.
Steps
- Utilized our CRM and SIS data to feed the model.
- Selected and implemented the most accurate machine learning model.
- Analyzed behavioral patterns to understand application variables.
Expanding upon the outcomes of this capstone project, plans for the next phase are underway. It involves the augmentation of the model with added machine learning techniques and the integration of a wider array of variables to refine the performance of our predictive analytics. This iterative refinement ensures that our recruitment strategies are not only informed by the most accurate data available but are perfectly aligned with our institution’s overarching goals.
The capstone project was enriched by a diverse array of resources. The key resource among these was EAB’s expertise and tools, coupled with the support from my institution. Reflecting on the journey, it has been a collaborative and resource-rich experience.
More Blogs
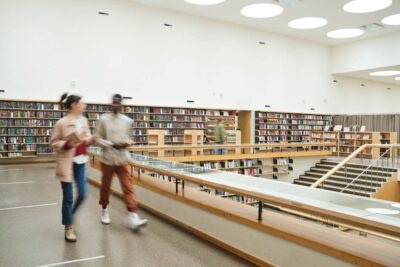
'No net new': What stuck with university leaders at our Campus Space Summit
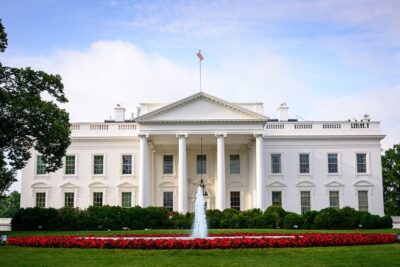
Responding to federal policy shocks: What we learned from nearly 50 higher ed institutions
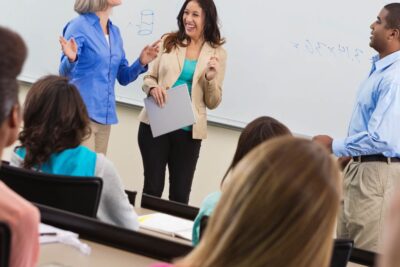