AI’s environmental impact can’t be ignored. What can higher ed do?
May 9, 2025, By Abhilash Panthagani, Associate Director, Strategic Research and Antoinette Waller, Research Analyst
AI is already an indispensable part of the higher education landscape. Students demand AI skills, faculty now compete for AI research grants, and campus leaders hold out hope that AI will bridge budget deficits. At the same time, some have concerns about AI’s environmental impact, pushing their institutions to confront the reality that the data centers running AI consume significant amounts of energy and water.
Colleges and universities must reconcile the need to adopt AI for its transformative potential with its environmental consequences. EAB has identified five takeaways to help your institution navigate growing campus concerns about AI’s impact on environmental sustainability.
1. AI environmental concerns are real, but the full extent of the impact is unclear
The most-cited statistics are startling: a single query on ChatGPT-4 uses ten times more electricity than a Google search; generating a 100-word email with GPT-4 uses a bottle of water. However, these figures are typically inconclusive, relying on napkin math from incomplete data.
What’s more, consumption estimates are constantly changing as models advance. In the context of everyday energy consumption, interpreting those figures becomes even less straightforward. For example, a laptop or LED lightbulb running for a few minutes consumes more energy than a single GPT-4 query.
Ultimately, the core environmental issue is not the energy cost of a query, but the explosive growth in AI usage driving the construction of data centers powered by fossil fuels instead of renewable energy. In the U.S. alone, data center capacity under construction surged by 70% from 2023 to 2024. And while many vendors are working to improve AI models and data center efficiency, rapid expansion remains their priority. Google, for example, reported that its emissions footprint rose by 48% since 2019, largely due to AI and data centers, while it only replenished 18% of the water it consumed.
2. Institutions should prioritize more energy-efficient AI options (when possible)
AI models continue to improve their energy efficiency. EpochAI, a nonprofit AI research institution, estimates that since ChatGPT launched, the energy used for each query has decreased tenfold. Techniques like combining parts of existing models (i.e., model merging) or activating just small parts of a model mean AI is getting greener without losing power.
DeepSeek dominated headlines in January 2025 after claiming it trained its R1 “reasoning model” for a fraction of the cost and computing power it took Meta to train its latest AI models. While some questions persist about how much more energy efficient these newer reasoning models are in action (not just to train) compared to older models, vendors are actively working to optimize AI models’ energy use.
3. Strategies to mitigate AI’s environmental impact depend on an institution’s position on the “build-versus-buy” spectrum
Institutions on the “build” side of the spectrum have greater direct influence over—and ability to mitigate —AI’s environmental impact. For example, when institutions host AI models on campus data centers (e.g., ASU’s CreateAI Platform), they are directly accountable for the associated energy use and emissions. However, they can also fully optimize both model and data center infrastructure for efficiency.
The following strategies align with an institution’s position along the build-versus-buy spectrum.
Build efficient data centers powered by clean energy when hosting AI on campus
An institution that chooses to host AI models or Application Programming Interfaces (APIs) using on-campus data centers (e.g., ASU’s CreateAI Platform) directly manages the associated energy consumption. As such, amid increased public scrutiny over AI’s environmental footprint, institutions should prioritize energy efficiency and clean energy when building or expanding data centers.
Unsurprisingly, leading research institutions that have historically spearheaded AI research have already begun making their data centers more sustainable:
- Harvard University’s Kempner AI Cluster is one of the fastest AI clusters in the world and operates entirely on carbon-free energy generated by solar arrays and a hydroelectric power station.
- MIT’s Lincoln Laboratory Supercomputing Center leads a number of projects to make computing more efficient, like enforcing a power cap to reduce hardware operating temperatures and training AI models when demand for local grid energy is low.
Prioritize energy-efficient products when customizing models or APIs in the cloud
Many institutions use open-source models (e.g., Meta’s LlaMa 4) or vendor APIs (e.g., OpenAI’s GPT-4.1 API) that run in the cloud to create custom AI applications. In these cases, the AI models still run in the vendor’s data centers, but institutions can directly influence how much energy the AI will consume by how they architect models and applications.
For example, they can use less powerful models for simpler tasks or group requests to an AI model (i.e., minimizing API calls). The goal should be to bring the most energy-efficient version of an AI application to production.
Demand vendor transparency when licensing AI tools
When licensing a vendor tool (whether AI or otherwise), the institution does not control the model or the associated energy consumed by the vendor’s data centers. Nevertheless, higher education can still influence outcomes by demanding greater sustainability transparency (and even action) from vendors.
Institutions can bring up these concerns in feedback sessions and when negotiating contracts, and even factor these considerations into purchasing decisions.
4. Faculty should be included in sustainability reviews of AI tool selection and development
Faculty are leading discussions around the ethical use of AI on campus. As such, some colleges and universities are convening groups like Arizona State University’s Faculty Ethics Committee on AI Technology to establish standards and review whether campus AI products adhere to ethical practices. This approach can be used to integrate sustainability considerations into the review of AI products and to stay informed as the discourse evolves.
5. Institutions can use the AI publicity as an opportunity to market and reenergize overall sustainability efforts
AI constitutes only a piece of an institution’s environmental impact. Many higher education institutions already publish sustainability plans and targets, while tracking progress with the Sustainability Tracking, Assessment & Rating System (STARS) and the United Nations’ Sustainable Development Goals. Finding ways to mitigate energy consumption and emissions is an ongoing effort for colleges and universities.
-
Advance your campus sustainability goals
Explore EAB’s Compendium of Sustainability Initiatives in Higher Education, a menu of over 100 sustainability initiatives, complete with prioritization guidance and higher ed case studies.
For example, institutions began purchasing carbon offsets for faculty travel a few years ago in response to growing outcry about travel-related emissions. The best argument a university can make is that it, as a whole and across all fronts, is taking sustainability seriously. This is more important than a singular stance on AI’s environmental impact. In the current climate, the goals your institution has and the measures it’s taking should be marketed to engender productive engagement in overall sustainability efforts.
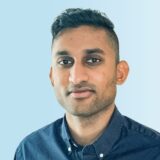
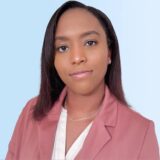
More Blogs
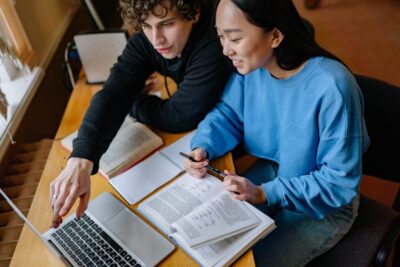
6 innovative ways higher ed can embrace AI
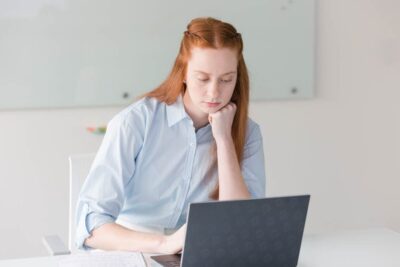