3 types of data every department chair needs (but usually doesn’t have)
July 27, 2018
Back in 2014, I worked with a team of researchers at the Gates Foundation to pinpoint main drivers of academic costs. We identified specific analyses that would empower department leaders to make smarter resource decisions.
Over the past few years, these analyses are even more relevant as more colleges and universities face state budget cuts, pressure on net tuition, lingering impacts of the Great Recession, increased demand for student services, and countless other factors that have forced both administrators and academic leadership to seek greater intentionality in resource allocation across campus.
But even as more schools embrace “efficiency” (previously a word not well received), resource allocation continues to be a top challenge for academic leaders. As one provost recently told me, “Making more intentional decisions on tricky questions like faculty workload, class size, target course completion rates, and program launches is easier said than done.”
The culprit: A lack of data and insights to build a foundation or blueprint to guide decision making. We’ll never have perfect data ahead of key decisions, but there are three categories of analysis that every college or university should be pursuing to optimize ever-scarce resources across campus:
1. Start conversations with academic program data
The missions of departments and programs aren’t static. In many cases, traditionally large departments, such as English and history, are shifting from churning out majors to churning out equally important general education coursework (teaching students from other programs). Getting ahead of trends like this, both on your own campus and at peer schools, enables leadership to make changes in future faculty and courses. For example, if a department with a decreasing proportion of credits taught to majors has an open faculty line or adjunct budget, you may hire someone who excels at teaching lower-division survey courses or perhaps focus on making sure enough 100- and 200-level sections are available to non-majors.
In addition to shifting departmental missions, it’s also critical to look at high-growth colleges and departments facing capacity constraints (e.g., full sections and relatively overburdened instructional staff). These departments are where you’d likely want to look first to expand resources. The flip side might be true as well: Shrinking departments with fewer capacity constraints might have to wait their turn for new faculty.
Conversations around change are never easy, but as one faculty senate president said, “We may not always get what we ask for, but as long as we understand the rationale, we feel respected.” And understanding the rationale means painting a more holistic picture: How does growth in one department affect other units that may not even be housed in the same college? The combination of unit-level student credit hours (SCH) tracking, plus an understanding of interdependency trends across campus, enables us to have smarter conversations about resource allocation.
Sample academic program analyses to inform resource allocation decisions:
- Relative growth of attempted student credit hours
- SCH taught by each department compared to the SCH taken by majors within departments
- Change in count of distinct course offerings within the department
2. Monitor student and course data
Keeping a pulse on student and course data gives academic leaders the ability to make adjustments to course offerings and faculty assignments. This ensures the right faculty are teaching the right students in the right types of courses. By monitoring DFW rates—the percentage of students who receive a failing grade of D or F, or withdraw from the course—and class size and fill rates, leaders can quickly determine where more resources are needed to support student progress.
Navigate the Bottleneck Courses at Your School
These data are particularly essential in managing to the “sweet spot” of section fill rates. You don’t want to routinely offer many under-filled sections of the same course, nor do you want to create “bottlenecks” by offering too few sections of an over-enrolled course. Aiming for that sweet spot—about 80%-90% full—requires a precise handle on your own institutional data, as well as a view into external benchmarks. For example, if your lower-division chemistry classes have under-filled sections over the last two terms, but benchmarks indicate growth in demand for similar classes at peer institutions, you may be less inclined to collapse them in a current term. These extrinsic demand benchmarks might reasonably indicate that we’ll grow into those additional sections.
Sample course data analyses to inform resource allocation:
- Class size distribution
- Course-level DFW rates
- Number of excess sections and bottleneck courses
4 Strategies to Reduce the Unproductive Credit Rate for Students
3. Examine faculty cost and workload data
Conversations about faculty workload and instructional costs are typically the third rail of academic management, but provosts and CBOs alike tell us that it’s time to pull the proverbial bandage off and have at it. Analysis of faculty and costs should focus less on a “standard teaching load” for faculty across an institution and instead more on benchmark instructional workloads at peer institutions in like departments. The range and median of workloads among peers, measured by sections or hours taught per term, are more instructive than an arbitrary standard.
Another key consideration in workload analysis is class size. If there is a path toward increasing median class size in, say, lower-division composition classes, that may free up some releases for full-time faculty. The typical (and sometimes fair) pushback in increasing class size is the potential negative impact on student outcomes—namely course-completion rates and pathway options. That’s why, when considering changes in class size, looking at benchmarks will help you determine if there is an incremental change that makes sense for your institution. If your lower-division English lectures capped at 18, but your peer’s departments have 20 students, it may be best to make an adjustment. And the resources you’ve made available in this example could be reallocated toward intentionally small classes.
Sample faculty and workload analyses to inform resource allocation:
- Department costs per student credit hour compared to peers
- Instructional full-time equivalency compared to number of instructional staff
More Blogs
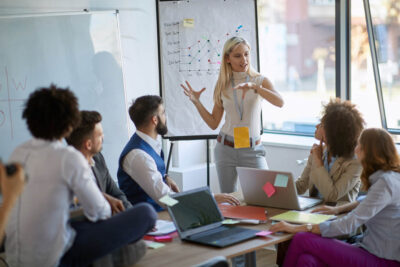
4 reasons your university should invest in a permanent process improvement team—and how to maximize its impact
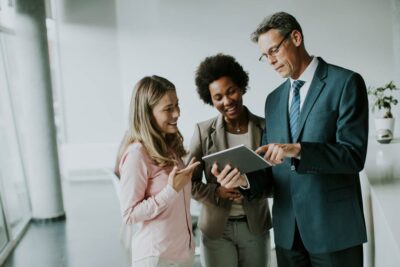
Higher education’s top cost savings opportunities
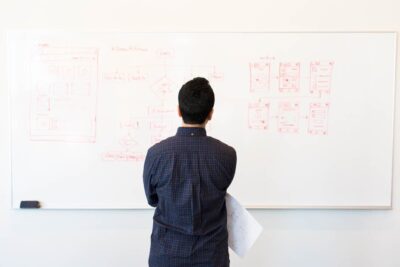