5 things campus leaders need to know about AI and machine learning
A primer on data science for higher ed executives
August 27, 2021, By Lars Waldo, Managing Director, Data Science
Following a long year of digital innovation in higher education, virtually all campus leaders have encountered new opportunities for campus data to help solve some of their institution’s most thorny problems. For some, the topic of artificial intelligence (AI) and machine learning (ML) may have surfaced in conversations across the board—from prospect outreach customization and student success interventions to better and more sustainable management of campus facilities. For others, proposals for using AI and ML models to help campus operations may be isolated to specific pockets: the IR or advising departments, a new vendor partner, or just a proactive employee with a passion project.
Unfortunately, understanding the needs, mechanics, and uses of AI/ML models requires specialized knowledge and experience, making it difficult to evaluate the pros and cons of any given system without an extensive technical background. And the stakes are high: if implemented poorly, or without a longer-term vision for support and actionability, data science investments risk setting institutions on the wrong course—or adding greater expense to operations without improving effectiveness.
To help executives make thoughtful recommendations on their decision-making journey, here are 5 things campus leaders must recognize before giving the green-light on a new data project:
1. Adopting AI/ML is a big commitment
Whether developed in-house or through partnership with a vendor, adopting AI/ML is a major commitment and it should not be undertaken without the institutional determination to see it through. Machine learning models are expensive and time-consuming to build because they rely on many hours of work from specialists who are experts in database technology, software engineering, and machine learning theory. Once trained, these models require ongoing upkeep and monitoring to make sure they are performing properly. In most cases, the people using or acting on the models are different from the people training them, so they need to be educated and convinced to use the output of the model appropriately.
Many applications of AI/ML—like raising graduation rates by supporting at-risk students—may take years to come to fruition. Patience, commitment, and leadership are needed at all stages of this process to benefit from AI/ML adoption.
2. Every model should lead to an action
Because AI/ML models are such a large commitment, it is important to make sure that every model your organization uses is tied directly to an action that can create a positive impact. Enrollment models should lead to better outreach campaigns. Student success models should lead to more strategic academic advising appointments. Course demand forecasts should trigger course scheduling reviews. You are investing lots of money or staff time into these models. If they are only producing interesting numbers, but not leading to actions or decisions, you are probably not getting good value from the model.
3. Data quality and integrity matter
“There are two interesting moments in the lifetime of a piece of data: the moment it is created and the moment it is used. Quality, the degree to which the data is fit for use, is judged at the moment of use,” writes Dr. Thomas Redman in the Harvard Business Review.
For higher education, so much of our data has been trapped or inaccessible in legacy systems for so long that one of the most prominent barriers to successful AI and ML projects is incomplete and inaccurate data. As the industry pushes toward new, more complex analytics for the purpose of increasing effectiveness and scaling customized student support, it is imperative to limit gaps, inaccuracies, and inconsistencies in your own data. Where data quality is poor, institutional trust will waver—and without trust in the models, there is no path from insight to action.
4. Winning over users can be the hardest part
One of the most common problems universities face when implementing AI/ML models has nothing to do with their technical or mathematical complexity – it has to do with getting the staff to understand, trust, and use the models. Depending on the model, the staff who are using it may have no technical background, may distrust AI/ML based on popular media depictions, or may be in a part of the organization that is distant and siloed from the technical people who built the model and under little-to-no pressure to make use of it. Whatever the cause of their concern, data deniers can quickly derail advanced analytics projects. Education, transparency, and organizational cooperation are critical to showing front-line staff that using AI/ML models to help make decisions is a safe, smart, and necessary part of doing their job.
5. AI/ML is part of the future on campus
As difficult as it can be to procure, implement, and operationalize AI/ML models, it isn’t an area that university leaders can afford to ignore. With enrollment in decline, students becoming more cost-conscious, and pressure mounting to focus on outcomes, AI/ML presents the opportunity to target prospects and students more effectively and run organizations more efficiently without the expense of adding staff to perform those duties. Furthermore, there are an ever-increasing number of on-campus systems collecting large amounts of data, which will allow for a tremendous proliferation of the areas of campus life that can benefit from the insights of AI/ML.
Campuses that take advantage of these opportunities and build their future atop an integrated Education Data Platform will pull ahead of the pack—and those that lag will see the gap to insight-driven strategies widening ahead of them.
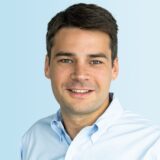
More Blogs
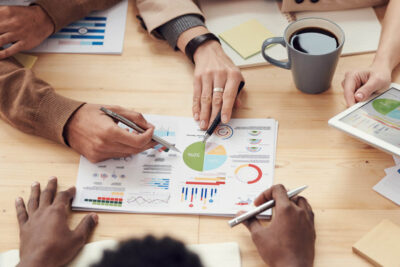
4 lessons on financial sustainability and organizational transformation in higher ed
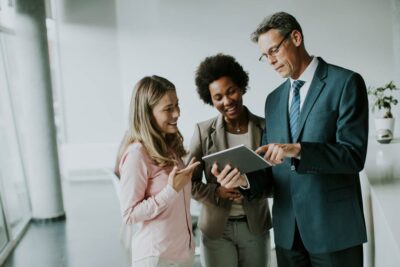
Higher education’s top cost savings opportunities
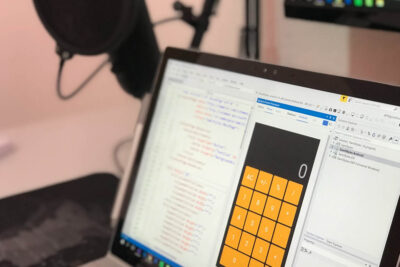