Is your data ready to support COVID-19 recovery?
June 29, 2020
COVID-19 hit higher education like a wrecking ball. Campuses closed their doors and remote learning bridged the gap to graduation. Meanwhile, the broader reach of the pandemic upended the economy and challenged some of our assumptions about work, life, and study—leaving individual campuses to adapt in real-time to the circumstances unfolding around them, and sending institutional data requests soaring.
Leaders looking to steer their campus through unchartered waters are relying on institutional and contextual data for crisis management, strategic planning, and decision support. But, as one former higher education CIO remarked publicly: “The one time in our lives we’ve actually needed ‘Big Data’, we don’t have it.”
While other industries have invested in data management platforms to build deep organizational and customer awareness through data, most colleges and universities continue to rely on ad-hoc analytics to solve their most pressing concerns. By most accounts, COVID-19 recovery will likely be a marathon, not a sprint—with change as the only constant—and an ad-hoc effort will burn out fast.
As analytics leaders prepare to support their institutions through these ongoing shifts, remedying any data shortcomings exposed in the crisis will build campus adaptability and ensure the long-term sustainability of campus transformation initiatives. To help you understand the efforts necessary on your campus, reflect on these three pillars of data readiness to guide your response: accessibility, quality, and agility.
1. Accessibility: Was our data ready when we needed it?
When new questions come up in a crisis, they’re usually urgent and more complex than the questions we’re used to answering. It often means tapping new data sources for analysis. But if data isn’t centrally managed, the process of integrating across the data-rich campus environment means pulling data from the systems it resides in and dealing with the system owners and vendor-specific data schemas of each source. Analysts must navigate the appropriate business logic to understand the data collected, and then standardize the information for analytics—often by working hand-in-hand with the various units gathering the data.
These processes all take time, and every moment wasted prolongs campus inaction. If your data isn’t easily accessible for analytics, it’s not serving the institution.
Is Your Team Overwhelmed with Ad Hoc Requests?
2. Quality: Was our data trusted by institutional leadership?
Once data is accessible and feeding live and ongoing decision-support, the question of quality is quick to emerge. With leaders relying on data to inform strategic interventions in the near term, rather than focusing on retrospective analyses, the integrity of available data is paramount. If data concerning student engagement, learning progress, and business operations are not widely trusted, analytics leaders will face challenges in supporting campus strategy.
Embedding quality improvements means collaborating across business units and data management leaders to process changes and technical validations to the data lifecycle. But it’s a necessary investment: if the cabinet can’t trust the data, analysis paralysis is going to set in fast.
Data Quality Begins with Good Data Governance
3. Agility: Was our data flexible enough to accommodate new questions?
Or stated more bluntly, did you have to “make do” with the data you had—even though it wasn’t quite the analysis that leadership was looking for?
For many institutions, longitudinal analysis and comparing past performance with present predicaments is hampered by the flattening of historical data. Transactional systems that override fields with new information and data warehouses that take nightly system snapshots create rigid and inflexible views of campus operations, and very few campuses have been able to invest the resources to create flexible data repositories.
Creating a campus-focused view of institutional data demands up-front investment in data modelling. It requires creating data objects that look across systems and retain the richness of historical information. If your analytics teams got pulled into complex data manipulation to support the COVID-19 response, it might be time to make an investment in data agility now, ahead of the questions of tomorrow.
More Blogs
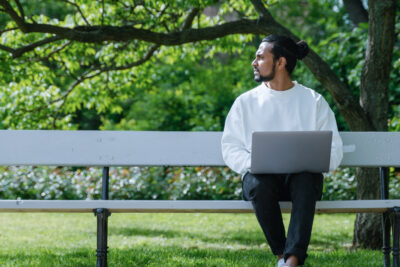
How can you tame data sprawl? Consider a chief data officer
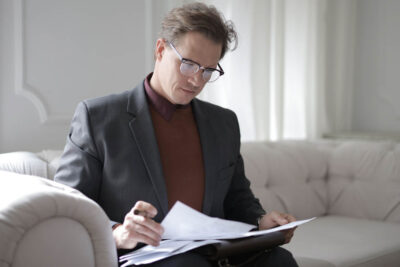
How to lead change when the change requires buying a new technology
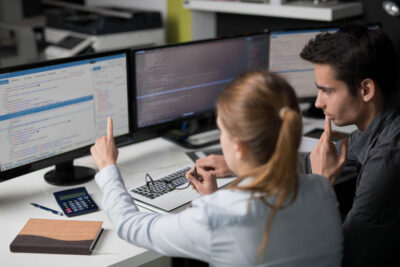