- All Blog Categories
- Adult Education Blog
- Advancement Blog
- Community College Blog
- Data & Analytics Blog
- Enrollment Blog
- Higher Education Strategy Blog
- K-12 District Leadership Blog
- Student Success Blog
Our Blogs
Get the latest insights from EAB experts on a variety of topics and terrains from enrollment to student success to K-12 education to higher education strategy and beyond.
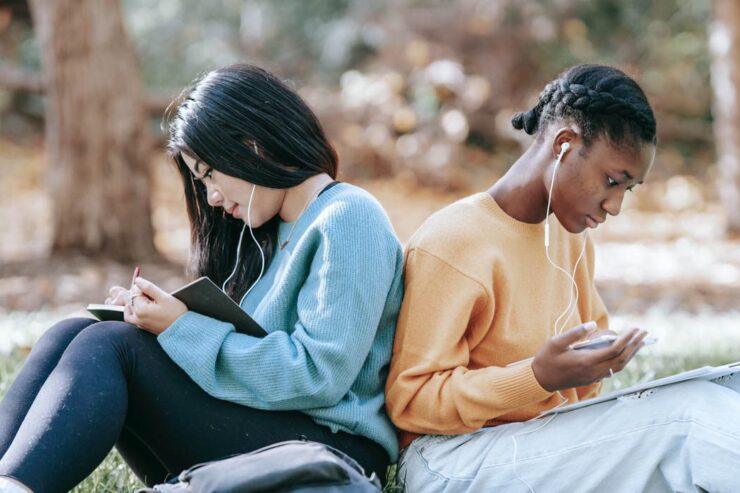
Are you prepared to recruit ‘Gen P’?
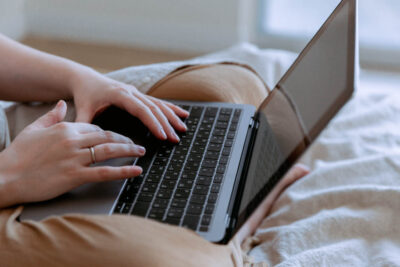
The top 5 ways prospective graduate and adult learners are finding your programs
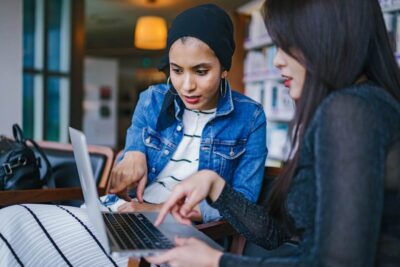
30 Student success priorities for the 2020s
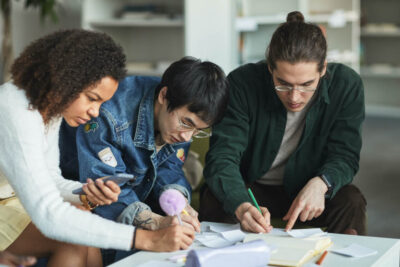
Most strategic plans fail to set useful goals. Why these mistakes make it impossible to meet institutional objectives.
EAB Blogs by the Numbers
100+
Blogs authored last year8
Different blogs that span topics in education53
Contributing expertsRecent and Featured Posts from Every Blog
-
Higher Education Strategy Blog
4 reasons your university should invest in a permanent process improvement team—and how to maximize its impact
Learn why dedicated process improvement teams are key to reducing inefficiencies and improving performance across campus.
Blog -
Adult Education Blog
Your digital marketing strategy is probably outdated—here’s how to fix it
If you’re still relying on outdated playbooks to identify high-intent graduate and adult learners, now’s the time to pivot. This blog breaks down what’s changed and what to do about it.
Blog -
Adult Education Blog
How graduate enrollment leaders—and prospective students—are using AI
AI isn’t just a passing fad. It is likely to be an integral part of how our society, and prospective students specifically, interact with marketing messages. To better understand how prospective graduate and adult learners perceive and use AI tools in their search process, our annual survey will dive deeper into these topics.
Blog -
Higher Education Strategy Blog
Eight things every provost should know about the K-12 math debate
To understand why math has been such a sticking point in academic recovery, we reached out to our K-12 partners to learn how to kickstart recovery before students reach college. Here’s what academic leaders need to know.
Blog -
Student Success Blog
What the OBBB and the new Carnegie classification could mean for higher education accountability
The new Carnegie classification and One Big Beautiful Bill both spotlight post-college earnings. Could this become the new benchmark for financial aid eligibility?
Blog -
Higher Education Strategy Blog
How is higher ed approaching DEI initiatives amid policy pressures?
Campus leaders shared how they're auditing their DEI initiatives in EAB interviews, a survey, and a listening session. Read the takeaways.
Blog -
Community College Blog
Four ways community colleges can improve career readiness
Discover 4 strategies community colleges can use to boost career readiness, close the skills gap, and help students succeed in today’s workforce.
Blog -
Higher Education Strategy Blog
'No net new': What stuck with university leaders at our Campus Space Summit
Read the blog for insights from our recent Campus Space Strategy Summit in London.
Blog -
Advancement Blog
4 ways advancement leaders can make the most of the summer
Learn 4 summer strategies to strengthen fundraising teams, improve donor engagement, build staff skills, and prepare for FY26 campaigns.
Blog
Great to see you today! What can I do for you?