- All Blog Categories
- Adult Education Blog
- Advancement Blog
- Community College Blog
- Data & Analytics Blog
- Enrollment Blog
- Higher Education Strategy Blog
- K-12 District Leadership Blog
- Student Success Blog
Our Blogs
Get the latest insights from EAB experts on a variety of topics and terrains from enrollment to student success to K-12 education to higher education strategy and beyond.
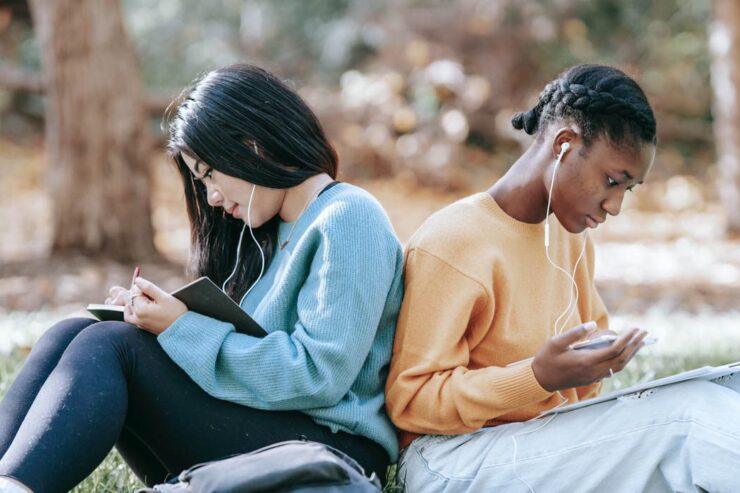
Are you prepared to recruit ‘Gen P’?
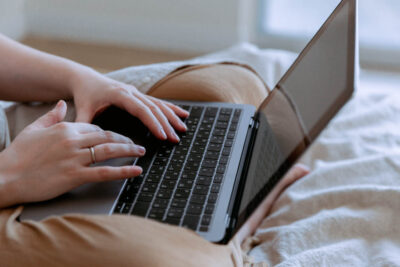
The top 5 ways prospective graduate and adult learners are finding your programs
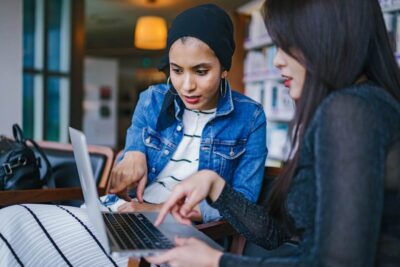
30 Student success priorities for the 2020s
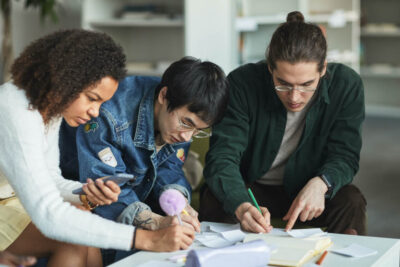
Most strategic plans fail to set useful goals. Why these mistakes make it impossible to meet institutional objectives.
EAB Blogs by the Numbers
100+
Blogs authored last year8
Different blogs that span topics in education53
Contributing expertsRecent and Featured Posts from Every Blog
-
Adult Education Blog
Is it time to transition away from your OPM?
Here’s a four-step framework for a smooth and strategic transition to a new enrollment marketing partner, without jeopardizing enrollment or revenue.
Blog -
Student Success Blog
Students need career support earlier. Here’s a scalable way colleges can provide it.
Blog -
Enrollment Blog
Brand management
Colleges and universities looking to win over prospective students have never faced a tougher environment, with strident critiques of higher ed having become commonplace in the media.
Blog -
Adult Education Blog
What it takes to win in grad and adult enrollment today
We asked four of EAB’s adult learner recruitment experts to share guidance on the biggest shifts affecting the graduate and adult enrollment field, and what enrollment teams can do to navigate them.
Blog -
Adult Education Blog
Our new staffing model playbook for higher ed CMOs
How can CMOs adapt their staffing models to meet today’s staffing and market realities? Here are three practices higher ed CMOs should consider.
Blog -
Higher Education Strategy Blog
4 reasons your university should invest in a permanent process improvement team—and how to maximize its impact
Learn why dedicated process improvement teams are key to reducing inefficiencies and improving performance across campus.
Blog -
Adult Education Blog
Your digital marketing strategy is probably outdated—here’s how to fix it
If you’re still relying on outdated playbooks to identify high-intent graduate and adult learners, now’s the time to pivot. This blog breaks down what’s changed and what to do about it.
Blog -
Adult Education Blog
How graduate enrollment leaders—and prospective students—are using AI
AI isn’t just a passing fad. It is likely to be an integral part of how our society, and prospective students specifically, interact with marketing messages. To better understand how prospective graduate and adult learners perceive and use AI tools in their search process, our annual survey will dive deeper into these topics.
Blog -
Higher Education Strategy Blog
Eight things every provost should know about the K-12 math debate
To understand why math has been such a sticking point in academic recovery, we reached out to our K-12 partners to learn how to kickstart recovery before students reach college. Here’s what academic leaders need to know.
Blog
Great to see you today! What can I do for you?