- All Blog Categories
- Adult Education Blog
- Advancement Blog
- Community College Blog
- Data & Analytics Blog
- Enrollment Blog
- K-12 Blog
- Strategy Blog
- Student Success Blog
Our Blogs
Get the latest insights from EAB experts on a variety of topics and terrains from enrollment to student success to K-12 education to higher education strategy and beyond.
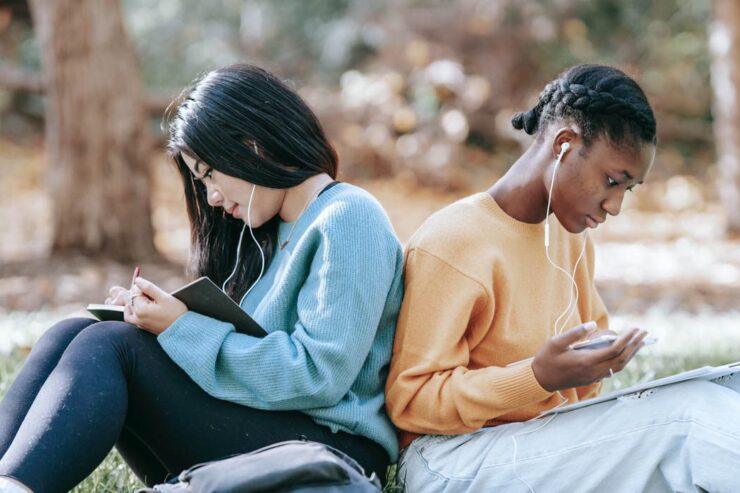
Are you prepared to recruit ‘Gen P’?
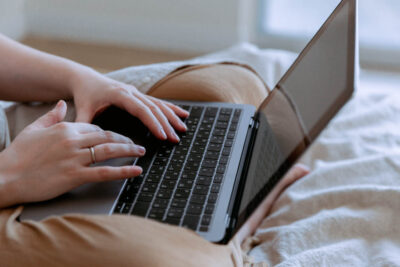
The top 5 ways prospective graduate and adult learners are finding your programs
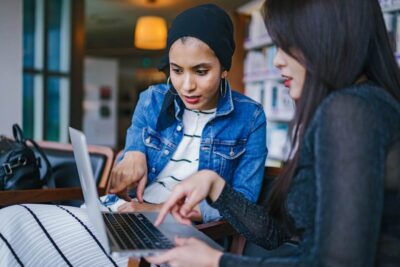
30 Student success priorities for the 2020s
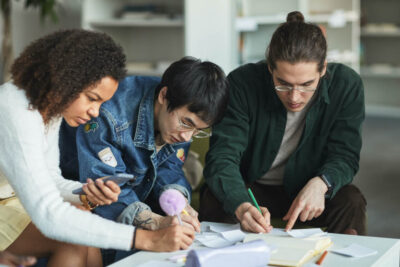
Most strategic plans fail to set useful goals. Why these mistakes make it impossible to meet institutional objectives.
EAB Blogs by the Numbers
100+
Blogs authored last year8
Different blogs that span topics in education53
Contributing expertsRecent and Featured Posts from Every Blog
-
Enrollment Blog
5 reasons not to neglect current high school juniors during yield season
In a typical spring, enrollment management teams have a laser-sharp focus on engaging admitted students. That has been even more pronounced in “unprecedented” years, such as the one we’re in right now, thanks to the botched FAFSA rollout and subsequent delays in financial aid awards and communications.
Blog -
Adult Education Blog
4 things graduate enrollment leaders want university presidents to know
Our EAB Adult Learner Recruitment team recently partnered with NAGAP, The Association for Graduate Enrollment Management, to conduct our annual surveys of graduate enrollment leaders around the country.
Blog -
Community College Blog
What is Guided Pathways—and is it still worth the hype?
Nearly 10 years after the term became ubiquitous, we provide a refresher on what Guided Pathways is, why technology is a critical component in scaling campus-wide efforts, and profile the most promising tech-focused practices from your peers.
Blog -
Advancement Blog
3 advancement challenges solved by digital marketing
It is no longer a question of whether to adopt digital strategies, but rather how to use them effectively. In this blog, we’ll define digital marketing and explore three ways digital marketing can strengthen your fundraising initiatives.
Blog -
Adult Education Blog
Tackle the three biggest challenges to your nursing program’s enrollment growth
Almost half of baccalaureate programs struggle to recruit faculty, and nursing programs at all levels struggle to secure clinical placements for their students, meaning your program likely faces significant barriers to long-term enrollment growth.
Blog -
K-12 Blog
Is your district adequately preparing students for a future with AI?
Learn how K-12 school districts can prepare students for a future with generative AI and how to navigate common AI barriers in education.
Blog -
Strategy Blog
4 conversations every president needs to have with their cabinet about name, image, and
likeness (NIL)EAB has identified four student-athlete enrollment questions presidents should ask their teams when talking about name, image, and likeness (NIL).
Blog -
Adult Education Blog
How to attract students across your entire education master’s program portfolio
In today’s market, many students are looking to advance their careers. Here are 3 marketing strategies to help you take advantage of this trend.
Blog -
Adult Education Blog
Graduate enrollment goals are increasing—here are 3 strategies to keep up.
From the much talked about demographic cliff to the proliferation of Gen Z in the grad and adult market, population and demographic changes are making the graduate market even more competitive. These market headwinds are impacting the enrollment goals that graduate education leaders set for themselves, as well as their team’s ability to meet these goals.
Blog